2024 Director’s Challenge Awards
This cycle, the program supported investigator-initiated, collaborative projects that use quantitative bioinformatics and/or computational approaches in biomedical and clinical research. The program made eleven 2-year awards, ranging in amount from $125,000 to $250,000 per year. The currently funded projects, project leaders, and collaborating Institute/Centers are:
- Discovering Inhibitors of Hemoglobin-S Polymerization in Silico & In Vitro
- Hans Ackerman in collaboration with NIDDK
- Prediction of Drug-Induced Liver Injury Using 3D Liver Tissue Models
- Marc Ferrer in collaboration with NIEHS
- Utilizing AI to Interpret Liver Histology and Predict Outcome in CHB
- Marc Ghany in collaboration with NCI
- Describing Pregnancy Physiology Using Data From Digital Research and Wearable Sensors
- Anne Marie Jukic in collaboration with NLM
- Machine Learning to Optimize Antibiotic Selection in Hospital Onset Sepsis
- Sameer Kadri in collaboration with NIAID, Uniformed Services University, University of Maryland, and George Washington University
- Multi-Omic Mechanistic Models to Identify Protective Dengue Immunity
- Leah Katzelnick in collaboration with CC
- Multiplex High Resolution Tissue Imaging of Metastatic Osteosarcoma
- Amy LeBlanc in collaboration with NIDDK
- TrialGPT 2.0: Boosting Efficiency in Trial Matching with AI
- Zhiyong Lu in collaboration with NCI, NICHD, and NEI
- An Artificial Intelligence Platform to Analyze Vaso-immune Architecture
- Dorian McGavern in collaboration with NIBIB
- CD22 Predicting Car T-Cell Toxicity & Response: A Bioinformatics-Based Approach
- Nirali Shah in collaboration with NIAID and CC
- Empowering Environmental Mixture Analysis through Novel Statistical Methods
- Shanshan Zhao in collaboration with NCI and NICHD
Discovering Inhibitors of Hemoglobin-S Polymerization in Silico & In Vitro
Principal Investigator (PI)
Hans Ackerman, M.D., D.Phil., M.Sc. (NIAID/DIR) in Collaboration with NIDDK
Project Summary
Sickle cell is an inherited blood disease that causes pain and anemia. If untreated, it leads to death at an early age. The sickle cell disease mutation causes deoxygenated hemoglobin-S (HbS) molecules to stick together and form long polymers, or fibers, that distort red blood cells into the sickle shape, blocking small vessels. Current drug treatments and curative therapies have significant drawbacks in terms of side effects or cost. What is needed right now is a drug that inhibits the sickling process directly to restore normal blood flow, ameliorate pain, and prevent organ injury. We propose the first computer-based screening to discover drugs that directly inhibit HbS polymerization. Advancements in computing power and structural bioinformatics, combined with the expansion of chemical libraries, provide an unprecedented opportunity to discover new drugs to treat sickle cell disease. We aim to (1) conduct virtual screening of compounds that bind to HbS and interfere with polymerization, (2) develop a new dynamic model of the HbS polymer in solution with which to refine our drug screen, and (3) test lead compounds in patients’ red blood cells in the lab.
This work will produce better drugs to treat sickle cell disease while advancing our understanding of protein self-assembly and aggregation. This will improve the lives of people living with sickle cell disease and help us to understand and treat a broad range of conditions from cancer to Alzheimer’s disease.
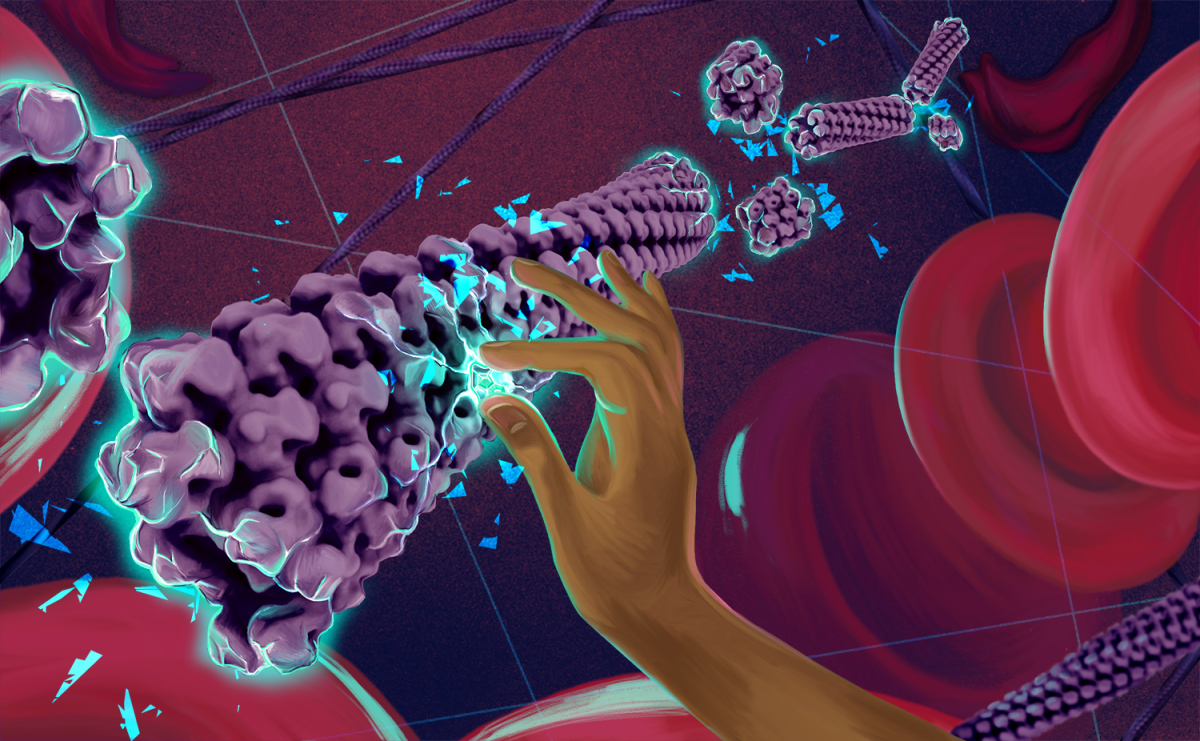
This original artwork by Rose Perry-Gottschalk (NIAID) conveys the approach and purpose of this collaborative project: to discover a drug that inhibits the polymerization of sickle hemoglobin (HbS). The sickle cell mutation creates new hydrophobic interactions between hemoglobin molecules that facilitates the polymerization of deoxygenated HbS into a multifilament helical fiber (shown in purple). The polymerization of hemoglobin causes red blood cells to form an abnormal “sickle” shape (shown in upper right), leading to obstruction of blood vessels, anemia, and pain. The shards of glass evoke patients’ descriptions of sickle cell pain while also representing our goal of shattering of the sickle cell fiber. We aim to identify a molecule that can disrupt the interactions between HbS molecules, break apart the sickle fiber, and restore red blood cells to their round shape. This goal is represented by the hand breaking the sickle fiber with this powerful yet elusive molecule.
Prediction of Drug-Induced Liver Injury Using 3D Liver Tissue Models
Principal Investigator (PI)
Marc Ferrer, Ph.D. (NCATS) in Collaboration with NIEHS
Project Summary
Drug-induced liver injury (DILI) is one of the leading causes of acute liver failure in the US and the most common reason cited for withdrawal of an approved drug. Most cases of DILI are from unexpected drug reactions not observed in animal models during development. While previous computational models have been built from in vitro data, their ability to predict DILI at required levels is lacking due to insufficient representation of human liver physiology. We propose a solution that combines the promise of novel three-dimensional (3D) tissue models with artificial intelligence/machine learning (AI/ML) techniques to establish a robust, predictive toxicology system that can protect against DILI elicited by drugs or environmental compounds. 3D tissue models can mimic essential physiological function of human tissues in response to xenobiotic exposures. We will assemble these 3D liver tissue models using primary human liver cells or liver cells derived from patient induced pluripotent stem cells (iPSCs). A set of 200 drugs with established associations to human DILI will be evaluated in these human 3D liver tissue models to generate multimodal data (including imaging, enzyme leakage, RNAseq, and metabolomics) that will train advanced AI/ML ensemble learners. The potential impact of this transdisciplinary proposal is a flexible approach for efficient generation of data to build robust computational predictions of DILI for drug development and precision environmental health.
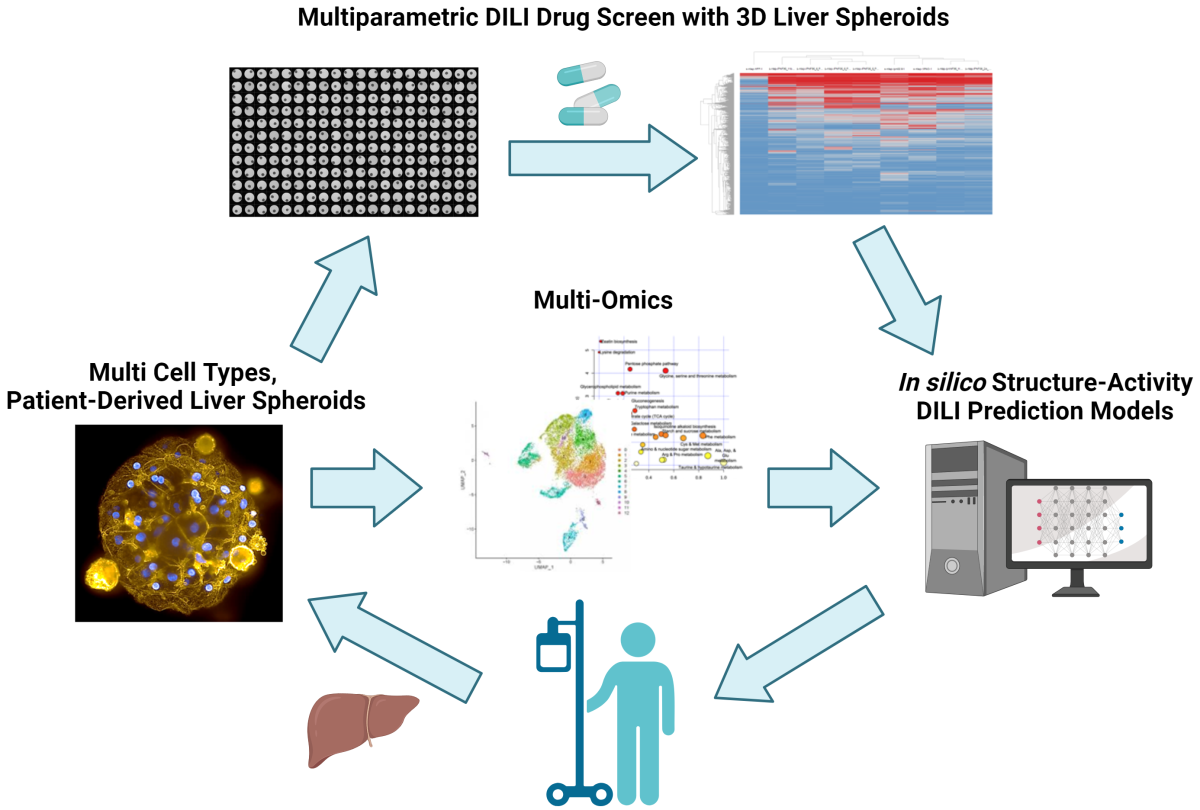
A combined in vitro/in silico platform of novel three-dimensional (3D) patient-derived liver tissue models with artificial intelligence/machine learning (AI/ML) techniques to establish a robust, predictive toxicology system that can protect against Drug-Induced Liver Injury (DILI) elicited by drugs or environmental compounds.
Utilizing AI to Interpret Liver Histology and Predict Outcome in CHB
Principal Investigator (PI)
Marc Gregory Ghany, M.D., M.H.Sc. (NIDDK) in Collaboration with NCI
Project Summary
Hepatitis B virus (HBV) is a hepatrophic virus that is associated with acute and chronic hepatitis. Almost 300 million people have chronic infection, resulting in ~900,000 deaths annually, mostly from complications of cirrhosis and hepatocellular carcinoma (HCC). Chronic hepatitis B is a very dynamic disease characterized by frequent shifts in disease activity which makes assessment of disease severity and prognosis challenging. Liver biopsy is regarded as the gold standard for assessing disease activity and severity and provides important prognostic information, but its interpretation is subject to inter-observer error. The development of whole slide imaging and refinements in artificial intelligence can be leveraged to identify patterns of pathology that can be used for risk stratification or prediction of disease outcome. Our goal is to utilize this technology to identify patterns of liver injury, using spatial distribution of inflammatory cells, coupled with single cell transcriptomics to identify specific immunological signatures associated with disease progression to predict clinical outcomes (progression to cirrhosis and development of HCC). An additional focus will be to evaluate changes in immunological signatures in response to antiviral therapy and link them to fibrosis regression and HCC risk. These investigations may provide mechanistic insights into development of HCC and identify new approaches to management and treatment.
Describing Pregnancy Physiology Using Data From Digital Research and Wearable Sensors
Principal Investigator (PI)
Anne Marie Jukic, Ph.D. (NIEHS) in Collaboration with NLM
Project Summary
The Apple Women’s Health Study (AWHS) is a digital, longitudinal study designed to gain a deeper understanding of variation in menstrual cycles and gynecologic conditions, including fertility, pregnancy, menopause and polycystic ovary syndrome (PCOS). Participants provide consent before data collection begins, participants choose what data they share with the AWHS, and a Certificate of Confidentiality provides additional privacy protections for the Study. Pregnancy and the outcome of pregnancy has been studied for decades, but the physiology underlying the changes during pregnancy is not well understood. Furthermore, risk factors for adverse pregnancy outcomes are not well-studied in large study populations that include diverse groups of participants. Prior studies have collected self-reported data on the onset of symptoms such as bleeding or cramping, but without markers of physiological changes. Identifying maternal physiologic responses during pregnancy would improve our biologic understanding and possibly provide identification of critical windows of high risk. The results of this project could inform future research on environmental exposures during more vulnerable windows of pregnancy. We will apply machine learning models to mobile application (app) health data aggregated through the Apple Women’s Health Study to better understand and identify the timing, occurrence, and risk factors for adverse pregnancy events

Machine Learning to Optimize Antibiotic Selection in Hospital Onset Sepsis
Principal Investigator (PI)
Sameer Kadri, M.D. (CC) in Collaboration with NIAID, Uniformed Services University, University of Maryland, and George Washington University
Project Summary
Hospital-acquired sepsis is a common cause of morbidity and mortality and is often caused by pathogens displaying antimicrobial resistance (AMR). Practice guidelines recommend patients with sepsis at risk of resistant pathogens promptly receive broader-spectrum empiric antibiotics. However, predicting AMR at time zero is challenging due to limited uptake of rapid AMR diagnostics and variable specificity of AMR risk factors. Consequently, clinicians frequently miscalculate AMR risk and prescribe either excessively broad or inadequate spectrum antibiotics, both of which can be detrimental. Machine learning (ML) has demonstrated success in improving AMR prediction accuracy. However, existing single center evaluations lack generalizability precluding uptake into daily workflow. Here, we will use de-identified electronic health records (EHR) from 1334 hospitals in Epic Cosmos database to develop an ML-based flexible framework for predicting AMR pathogens in hospital-onset sepsis. Nationally distributed data and AMR epidemiology will enhance generalizability and real-world utility. Optimal algorithms will be calibrated to unique and underrepresented populations and externally validated at 12 representative University of Maryland hospitals. Here, we will also test whether prediction is enhanced by natural language processing of unstructured notes and results. Eventually, a pragmatic clinical trial will test impacts of EHR-deployed ML algorithms on antibiotic selection and outcome.
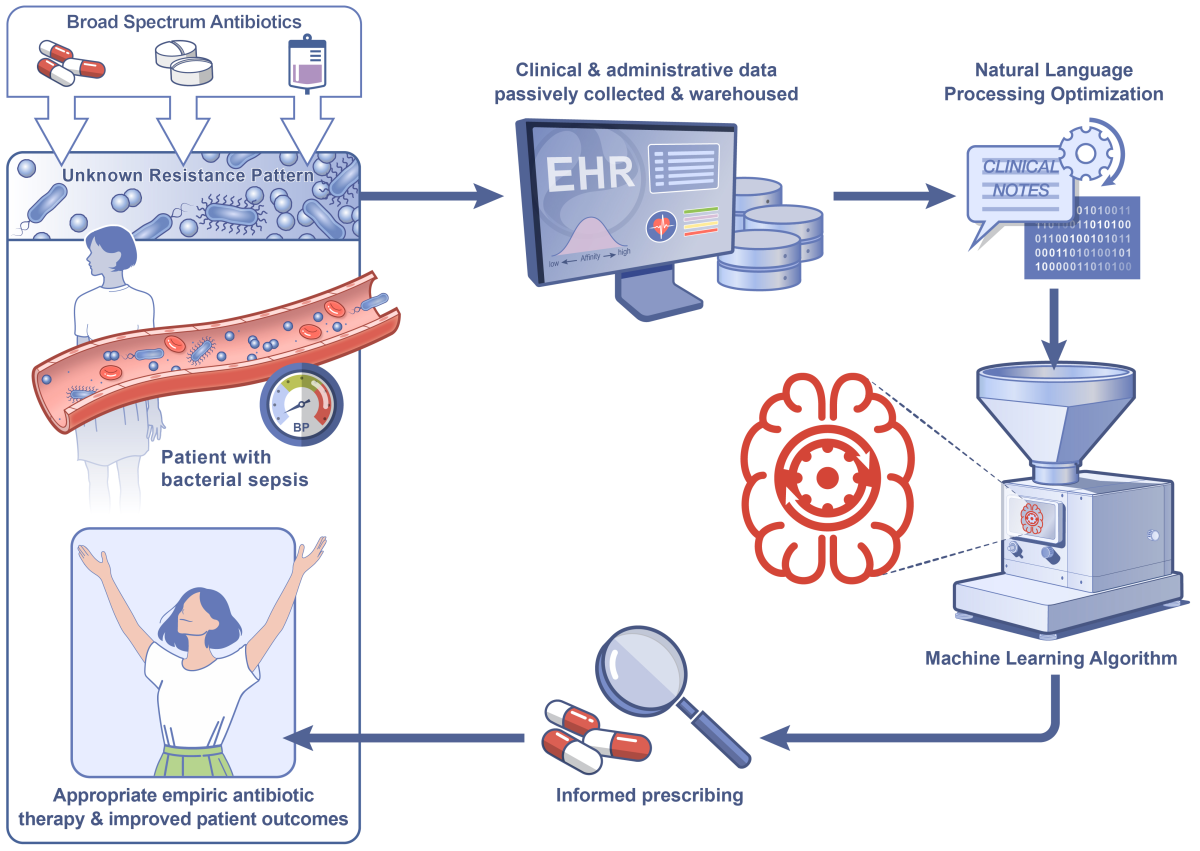
A schematic diagram detailing the proposed strategy of applying machine learning to structured and unstructured data elements from electronic health records to predict antimicrobial resistant pathogens and optimize empiric antibiotic selection in patients presenting with hospital-onset sepsis.
Multi-Omic Mechanistic Models to Identify Protective Dengue Immunity
Principal Investigator (PI)
Leah Katzelnick, Ph.D., M.P.H. (NIAID/DIR) in Collaboration with CC
Project Summary
Emerging diseases including dengue fever present a major threat to global health. Inadequate efficacy of licensed dengue vaccines is a major concern. In this proposal, we will:1) develop multi-scale mechanistic mod-els of host-viral dynamics and integrative modeling with multi-omics data to elucidate differential molecular and cellular factors underlying responses to dengue virus infection and vaccination, and 2) use clinical and experi-mental data to train predictive models for optimal identification, and sampling of the draining lymph nodes (LNs) in vaccinated and infected individuals. To characterize factors explaining vaccine responses and long-term broad protection, we will formulate mechanistic mathematical models calibrated using the unique clinical and multi-omics data collected in our ongoing dengue vaccine trial of 45 individuals across eight timepoints, including peripheral blood and LN samples. Collaboration with Interventional Radiology at the NIH Clinical Center on our trial facilitates optimized sampling of the LNs and enable investigation of the dynamics and specificity of germinal center B cells in humans. We will employ and cross-validate machine learning algorithms to predict successful LN sampling using features collected during the procedure and sample processing. The results of this multidisciplinary research will identify novel features of disease pathogenesis and broad protective immunity, advancing development of effective vaccines and treatments.
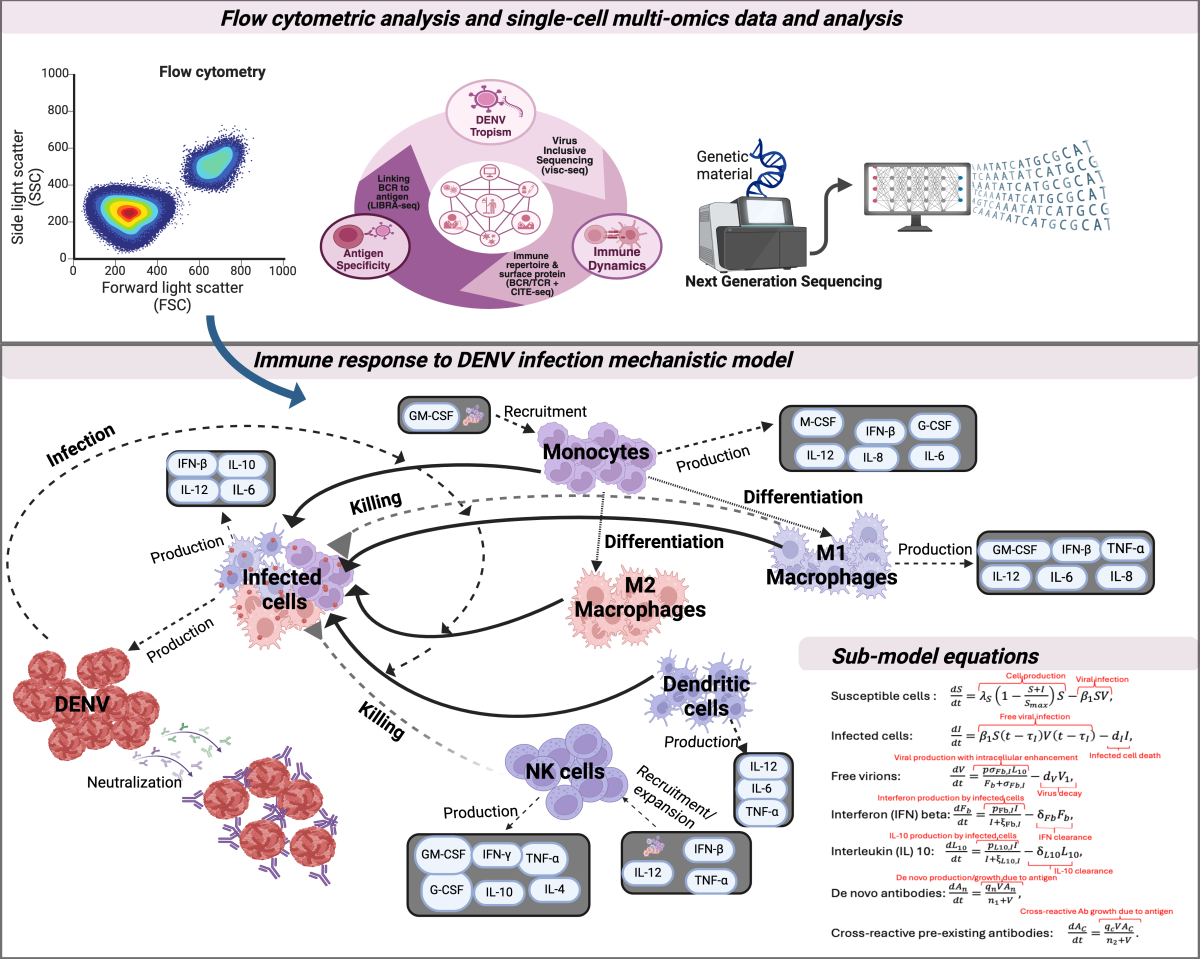
Schematic of the pipeline for in-depth characterization of broad protective immunity after primary, secondary and tertiary dengue virus (DENV) exposure. Cellular measures of immunological activity and multimodal single cell immune profiling data including bioinformatics analysis (upper panel). Mechanistic model framework of immune response to DENV infection and accompanying differential equations for the immune interactions (lower panel). Figure created with BioRender.com.
Multiplex High Resolution Tissue Imaging of Metastatic Osteosarcoma
Principal Investigator (PI)
Amy LeBlanc, D.V.M. (NCI/CCR) in Collaboration with NIDDK
Project Summary
Osteosarcoma is a rare, highly metastatic bone tumor of children and adolescents for which significant advances in care have not occurred in over 40 years. Tumor spread, or metastasis, is a complex, poorly understood and life-limiting aspect of osteosarcoma, and for which few effective therapies exist. Animal models - typically involving mice - are highly valuable for testing new approaches to treat osteosarcoma, but it remains unclear which models are the most informative for study of metastasis. Pet dogs naturally develop osteosarcoma at a 10-fold higher rate than humans and because of their cancers' close resemblance to humans, routinely participate in clinical trials designed to combat metastasis. In this proposal, we leverage the power of multiplex cleared tissue light-sheet microscopy and three-dimensional reconstruction for visualizing and contrasting critical structures and cell populations that exist in and around osteosarcoma metastases from mouse models, pet dogs, and human patients. This approach will enable, for the first time, cross-species exploration of metastatic tumors and their surrounding microenvironmental structures at a subcellular level in three-dimensional space. Such data will support selection of appropriate models for testing of new therapies destined for human use and will provide critical information on how closely naturally-occurring canine osteosarcoma vs. experimentally-induced metastatic osteosarcoma in mice recapitulate human disease.
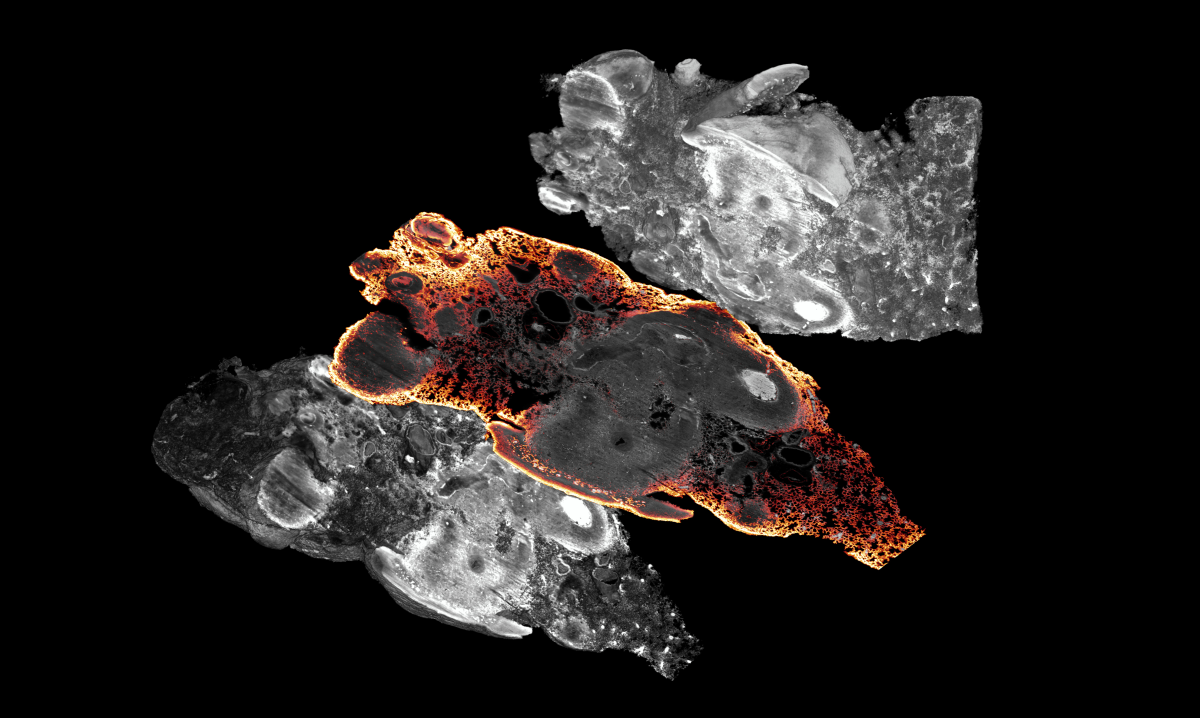
Mouse lung with metastatic osteosarcoma contained within, stained with a CD31 antibody to highlight the vasculature of the lung and around the tumor nodules.
TrialGPT 2.0: Boosting Efficiency in Trial Matching with AI
Principal Investigator (PI)
Zhiyong Lu, Ph.D. (NLM/NCBI) in Collaboration with NCI, NICHD, and NEI
Project Summary
Clinical trials are vital the development of novel treatments, yet patient recruitment remains a significant challenge. Traditional methods of manually reviewing patient histories against trial criteria are labor-intensive and pose a barrier to access that contributes to disparities in clinical trial enrollment. While machine learning and AI techniques have shown potential in matching patient data with clinical trials, their practical application has been hindered by the scarcity of training data. Recent advances in large language models (LLMs) have demonstrated exceptional performance in a variety of tasks with minimal data requirements. We have developed a proof-of-concept system named TrialGPT that utilizes LLMs to efficiently match patients with suitable clinical trials. We propose to further enhance TrialGPT by incorporating a chat interface that identifies information gaps and proactively resolves them through user interaction. Additionally, we will expand its application to real-world patient data and clinical trials across multiple Institutes/Centers (ICs). This approach is expected to significantly boost the efficiency of patient recruitment. Finally, this work would establish the feasibility of applying TrialGPT to extramural clinical trials and enable initial testing directly with key stakeholders within a secure environment.
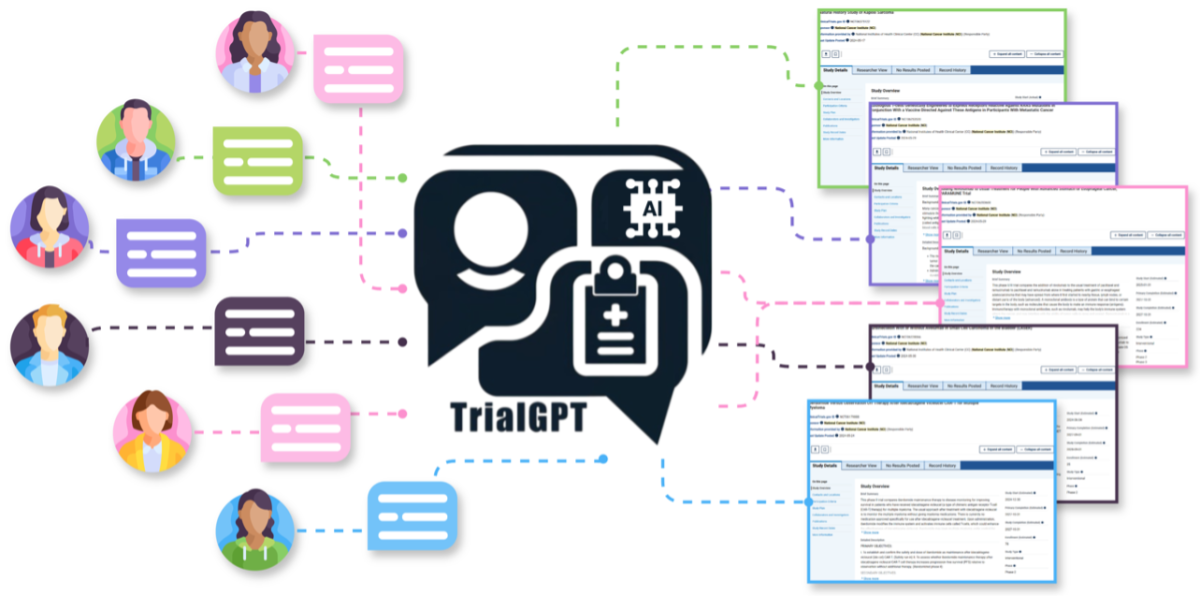
Proposed Overall Framework for Effective Patient-to-Trial Matching using Generative AI.
An Artificial Intelligence Platform to Analyze Vaso-immune Architecture
Principal Investigator (PI)
Dorian McGavern, Ph.D. (NINDS) in Collaboration with NIBIB
Project Summary
The CNS contains a complex labyrinth of blood vessels that vary in composition and architecture. For example, vessels residing in CNS barrier structures possess openings that allow peripheral communication, whereas those found in brain tissue are tightly sealed. Maintenance of CNS vasculature is required to preserve neural homeostasis, and deterioration contributes to the neurological decline observed during aging, neurodegenerative diseases, traumatic brain injuries, strokes, infections, and autoimmune diseases. Blood vessels also have a crucial role in directing all CNS immune activity. Given this level of complexity, there is still much to learn about the CNS vaso-immune landscape, and we believe this exciting area of research is highly relevant to our understanding of neural health and most neurological conditions. For this project, we have assembled a team of experts in CNS vasculature, advanced imaging, and artificial intelligence. Our goal is to generate the first high resolution atlas of vasculature and innate immune cells in the intact skull, meninges, and cortex of a mouse, and then train a neural network to recognize relevant changes in this atlas that occur after vascular remodeling or during disease. We will also develop a novel AI platform to analyze the massive (multi-terabyte) high resolution images that we generate. Development of this platform will represent a major advance in the field and solve a “big data” analysis problem faced by all biomedical imagers.

3D visualization of cerebrovasculature. This 3D image captured using a lightsheet confocal microscope shows vasculature in the cleared bone, meninges, and brain of a mouse.
CD22 Predicting Car T-Cell Toxicity & Response: A Bioinformatics-Based Approach
Principal Investigator (PI)
Nirali Shah, M.D., M.H.Sc. (NCI/CCR) in Collaboration with NIAID and CC
Project Summary
Chimeric antigen receptor (CAR) T-cell therapy has been transformative in treating patients with relapsed/refractory leukemias. As one of the first centers to test CAR T-cells in children and young adults, we have treated over 180 patients since 2012, primarily for B-acute lymphoblastic leukemia. Accordingly, we have amongst the most extensive clinically annotated datasets linked to key outcomes and a wealth of samples which can be used for comprehensive analysis to identify factors associated with toxicity and response. Importantly, this includes serial peripheral blood and bone marrow sampling from pre- and post-treatment timepoints, surplus apheresis products, and excess CAR T-cell products on nearly all patients. Building upon pre-existing trans-NIH intramural collaborations to explore factors associated with key outcomes, we aim to utilize an innovative systems biology-based approach, incorporating multiple hierarchies of information ranging from patient based clinical factors, to CAR T-cell engineering variables, to disease/tumor microenvironment single-cell biological signatures, with the goal of integrating this information together to understand the complexity of CAR T-cell related toxicity and response. A systems biology approach is essential to understanding CAR T-cell therapy outcomes as the treatment itself is derived directly from the patient with active disease, highlighting the importance of understanding patient specific biology at multiple levels.

Using a bioinformatics-based approach to predict toxicity and response to CAR T-cells.
Empowering Environmental Mixture Analysis through Novel Statistical Methods
Principal Investigator (PI)
Shanshan Zhao, Ph.D. (NIEHS) in Collaboration with NCI and NICHD
Project Summary
Humans interact with a mixture of environmental exposures daily. These exposures are known to play important roles in the etiology of many diseases, including cancer, reproductive disorders, respiratory and autoimmune diseases, and can serve as strong predictors of disease incidence and progression. Recent methodologic advances enable researchers to analyze them simultaneously to reveal the complex relationship between mixtures and health outcomes, which is superior to focusing on single, isolated exposures. Carefully designed population-based studies and sophisticated statistical/computational tools are needed for this purpose. We propose an extensive and focused collaborative effort from a group of PIs across ICs (NIEHS, NCI, NICHD) and disciplines (biostatistics, epidemiology, toxicology) who have made individual progress in mixtures research, to provide critical evaluations of statistical and computational tools for mixtures data and to develop methods directly relevant to IRP research, through the NIH Director's Challenge Innovation Award. We specifically propose to focus on three fundamental challenges for mixtures analysis, including handling incomplete exposure measurements due to detection limits in chemical assays, incorporating scientific knowledge of mixtures into disease etiology, and developing statistical learning methods for mixtures. The environment of the NIH IRP and support from this award are essential for us to make unique contributions to this field.
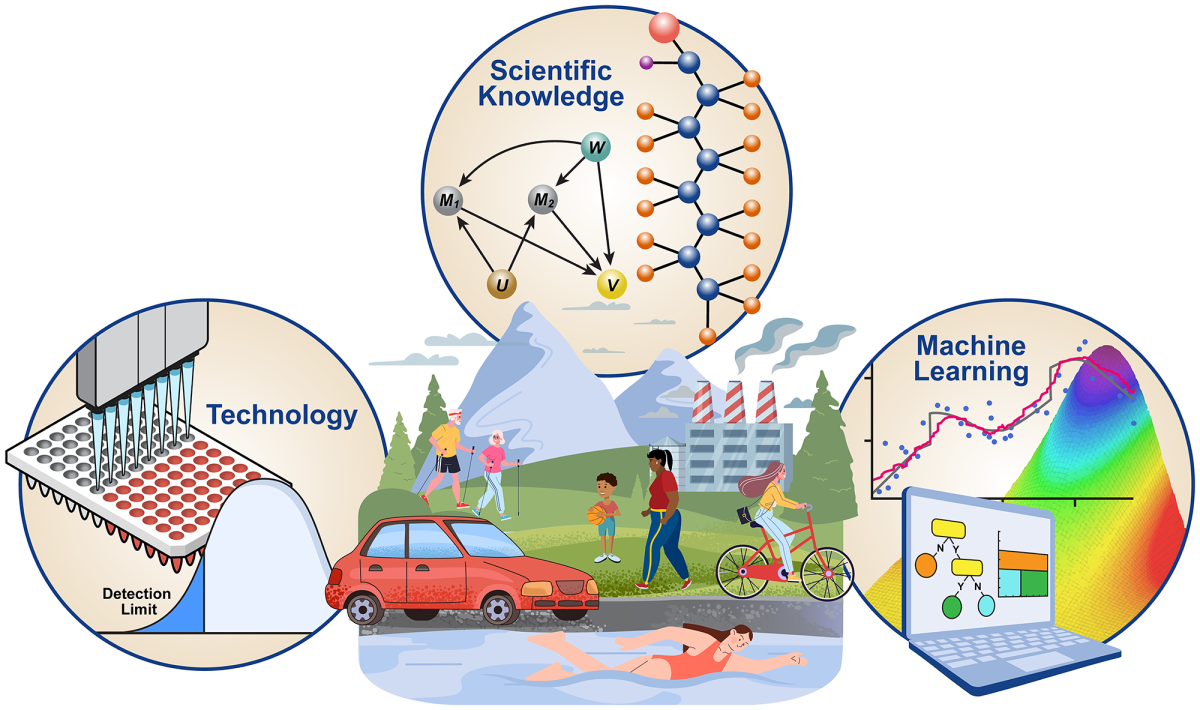
Empowering environmental mixtures analysis through innovative statistical methods, to handle incomplete exposure measurements due to technology limitations, to incorporate scientific knowledge, and to facilitate new discoveries with statistical learning methods.
This page was last updated on Thursday, February 20, 2025