How Neuromorphic Computing Can Help Us Understand the Brain
to
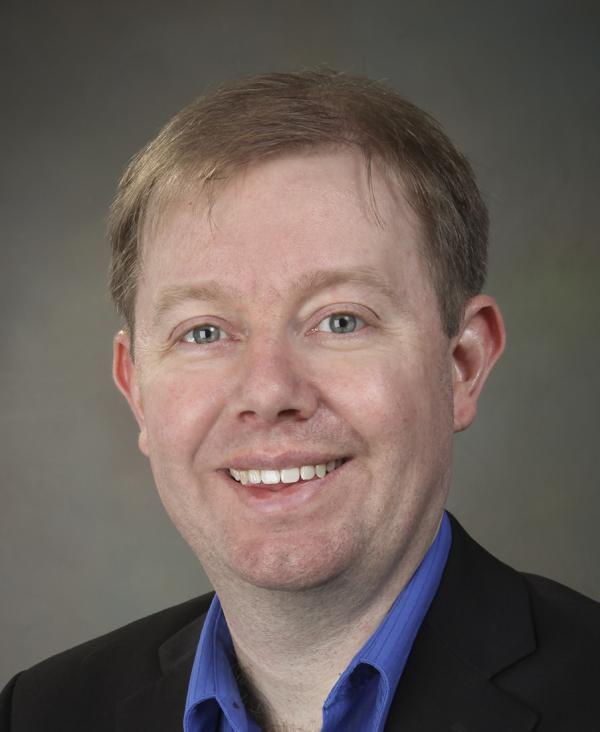
James Bradley Aimone, Ph.D.
Distinguished Member of Technical Staff, R&D Computer Science
Sandia National Laboratories
Dr. Brad Aimone is a Distinguished Member of Technical Staff in the Center for Computing Research at Sandia National Laboratories, where he is a lead researcher in leveraging computational neuroscience to advance artificial intelligence and in using neuromorphic computing platforms for future scientific computing applications. Brad currently leads a multi-institution DOE Office of Science Microelectronics Co-Design project titled COINFLIPS (which stands for CO-designed Influenced Neural Foundations Inspired by Physical Stochasticity) which is focused on developing a novel probabilistic neuromorphic computing platform. He also currently leads several other research efforts on designing neural algorithms for scientific computing applications and neuromorphic machine learning implementations.
Brad has published over seventy peer-reviewed journal and conference articles in venues such as Advanced Materials, Neuron, Nature Neuroscience, Nature Electronics, Communications of the ACM, and PNAS and he is one of the co-founders of the Neuro-Inspired Computational Elements, or NICE, conference. Prior to joining the technical staff at Sandia in 2011, Dr. Aimone was a postdoctoral research associate at the Salk Institute for Biological Studies, with a Ph.D. in computational neuroscience from the University of California, San Diego and Bachelor’s and Master’s degrees in chemical engineering from Rice University.
Summary
An underappreciated challenge of understanding the health implications of neural dynamics is the wide range of temporal and spatial scales in the brain. Neurological and mental health disorders often manifest themselves over months and years, while most neuroscience research focuses on dynamics over shorter timescales. This is especially a challenge for computational approaches, in which neural simulations are often limited to several seconds due to compute costs, greatly limiting their use for understanding fundamental neuroscience and health.
Inspired by my experiences in modeling adult hippocampal neurogenesis, in this talk I will describe how the challenges of modeling the diverse time and spatial scales of the brain’s plasticity and dynamics led me to look towards the emerging field of neuromorphic computing as a potential solution. Today’s neuromorphic systems are beginning to reach brain-like scales in terms of numbers of neurons. I will describe a range of unexpected results that we have learned from brain-like computing systems operating at this scale on a wide range of cognitive and non-cognitive tasks. In addition to providing a tool to perform neural simulations, neuromorphic hardware may enable energy-efficient artificial intelligence. Moreover, neuromorphic approaches offer a path towards more advanced real-time computing capabilities for biomedical applications with strict performance, efficiency, and security demands. Finally, I will revisit my original goal of how understanding neural computations at biologically realistic scales on neuromorphic systems can, in turn, help us understand how and why the brain operates the way that it does.
Learning Objectives
- Educate NIH community on today’s state of brain-inspired computing, specifically neuromorphic computing
- Describe how neuromorphic computing can bring brain-scale modeling to drive neuroscience theory in new directions
- Highlight the engineering benefits of neuromorphic computing (e.g., low power, learning, dynamics, etc.) that can be used to improve biomedical and AI-for-health applications.
https://videocast.nih.gov/watch=55007
This page was last updated on Thursday, October 10, 2024